Self-Instruct
SELF-INSTRUCT: Aligning Language Models with Self-Generated Instructions
使用LLM自动从已有的task instructions生成一系列新的task instructions进行instruction
-tuning。ACL 2023,华盛顿大学,代码。
Large “instruction-tuned” language models (i.e., finetuned to respond to instructions) have demonstrated a remarkable ability to generalize zero-shot to new tasks. Nevertheless, they depend heavily on human-written instruction data that is often limited in quantity, diversity, and creativity, therefore hindering the generality of the tuned model. We introduce SELF-INSTRUCT, a framework for improving the instruction-following capabilities of pretrained language models by bootstrapping off their own generations. Our pipeline generates instructions, input, and output samples from a language model, then filters invalid or similar ones before using them to finetune the original model. Applying our method to the vanilla GPT3, we demonstrate a 33% absolute improvement over the original model on SUPER-NATURALINSTRUCTIONS, on par with the performance of InstructGPT 001, which was trained with private user data and human annotations. For further evaluation, we curate a set of expert-written instructions for novel tasks, and show through human evaluation that tuning GPT3 with SELF-INSTRUCT outperforms using existing public instruction datasets by a large margin, leaving only a 5% absolute gap behind InstructGPT 001 . SELF-INSTRUCT provides an almost annotation-free method for aligning pretrained language models with instructions, and we release our large synthetic dataset to facilitate future studies on instruction tuning.
1. Introduction
人工生成instructions一方面代价很大,另一方面人工生成的instructions难以保证quantity, diversity, and creativity。
作者提出使用LLM从已有的task instruction出发,自动生成新的task instruction和对应的input-output,然后过滤掉不符合规则的新task instructions,再加入到已有的task instructions集合中。作者在这个自动构造的instruction data上fine-tuning GPT3,发现效果提升了33%,非常接近InstructGPT001的效果。
2. Method
作者提出的方法:
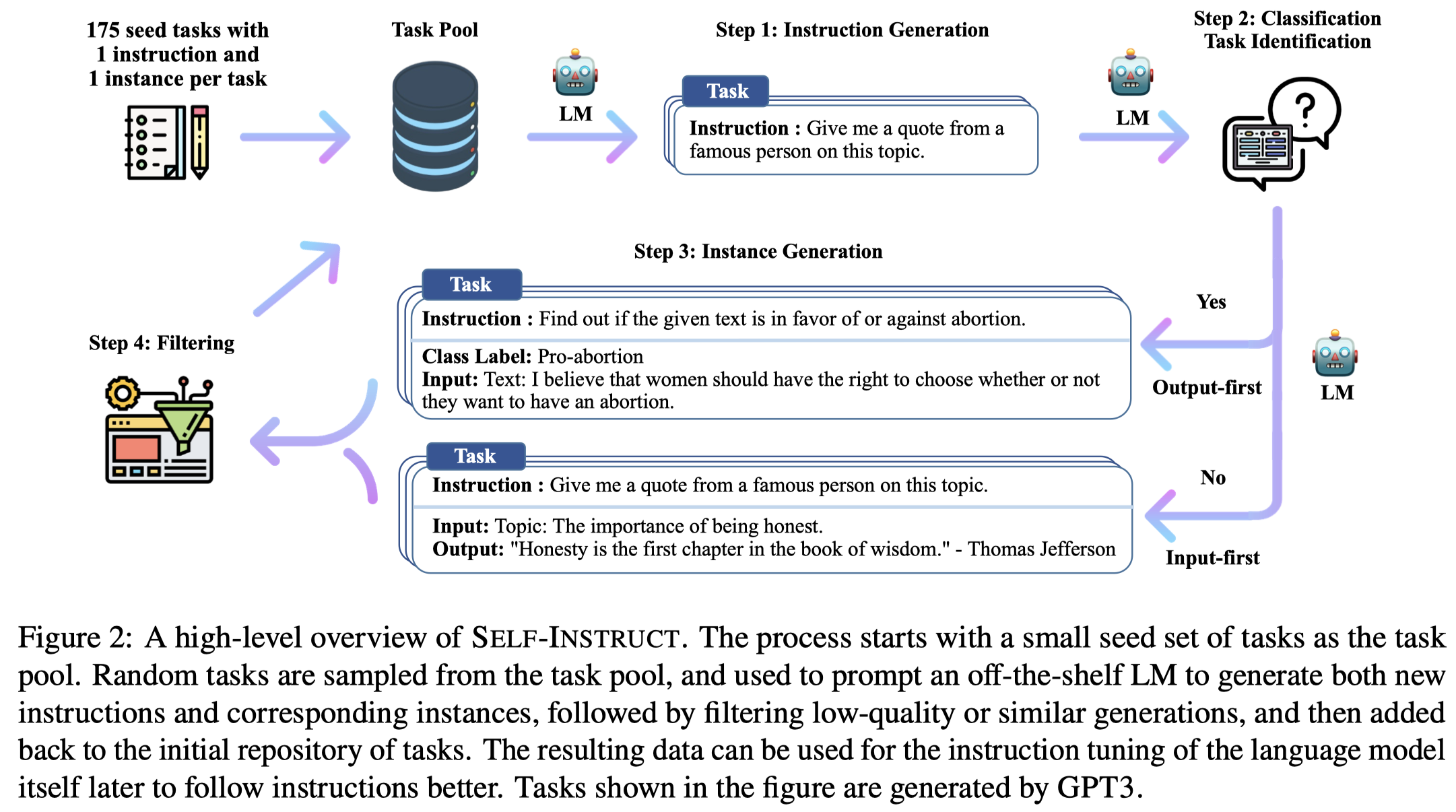
首先,作者拥有一个task pool,包括175 tasks (1 instruction and 1 instance for each task)。这175个初始的task instructions都是由本文作者自己创建的。
然后,作者从task pool中随机抽取8个task instructions(6 are from the human-written tasks, and 2 are from the model-generated tasks)。下面是产生新task instruction的prompt:
之后,作者使用LLM判断新产生的instruction是否是一个classification task(using 12 classification instructions and 19 non-classification instructions):
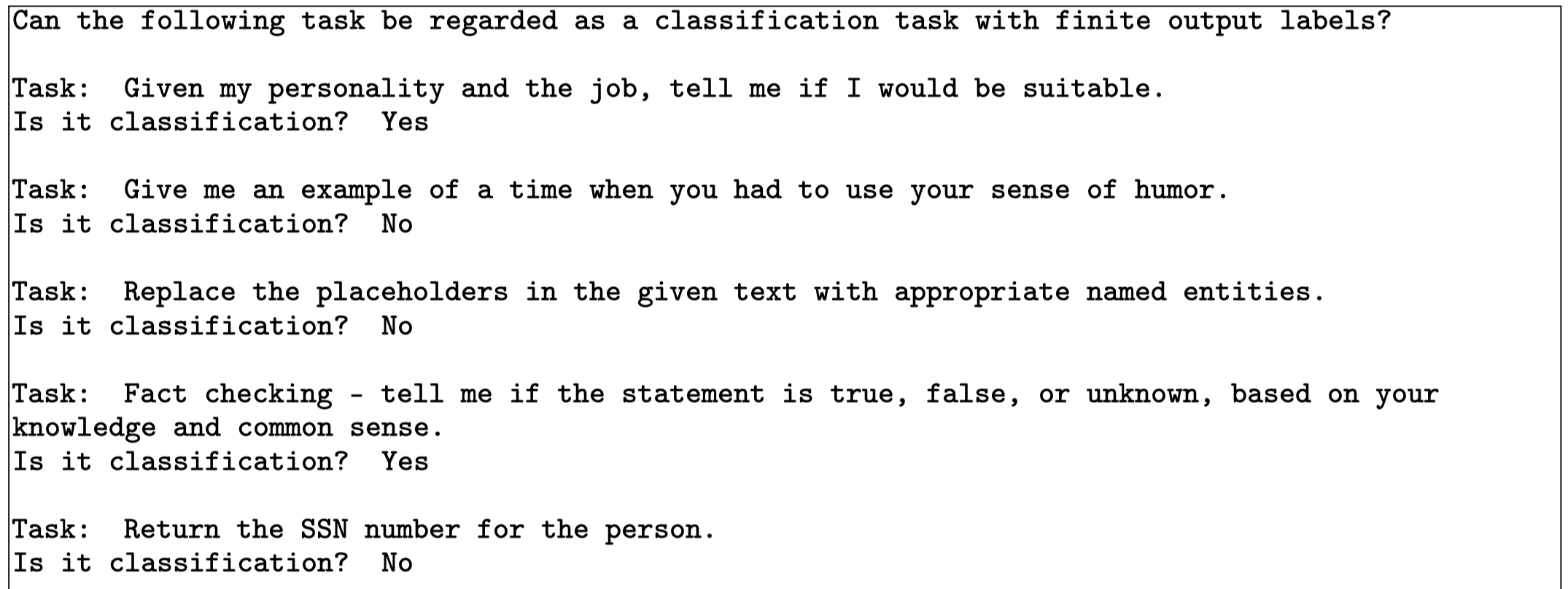
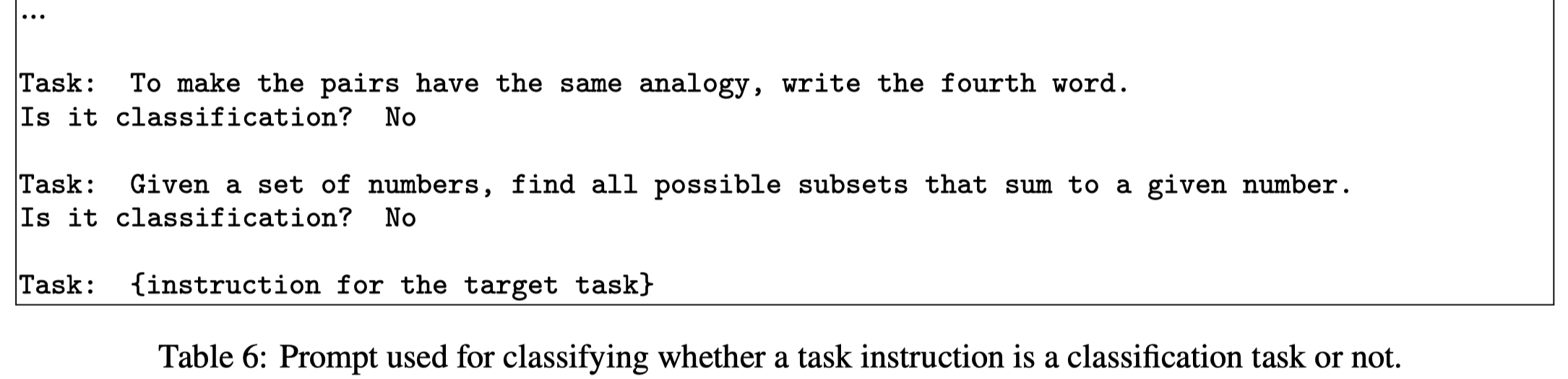
随后,对于新产生的task instruction,用LLM生成新的对应的instance。对于生成任务,作者先生成input,再生成output,作者称为Input-first Approach:
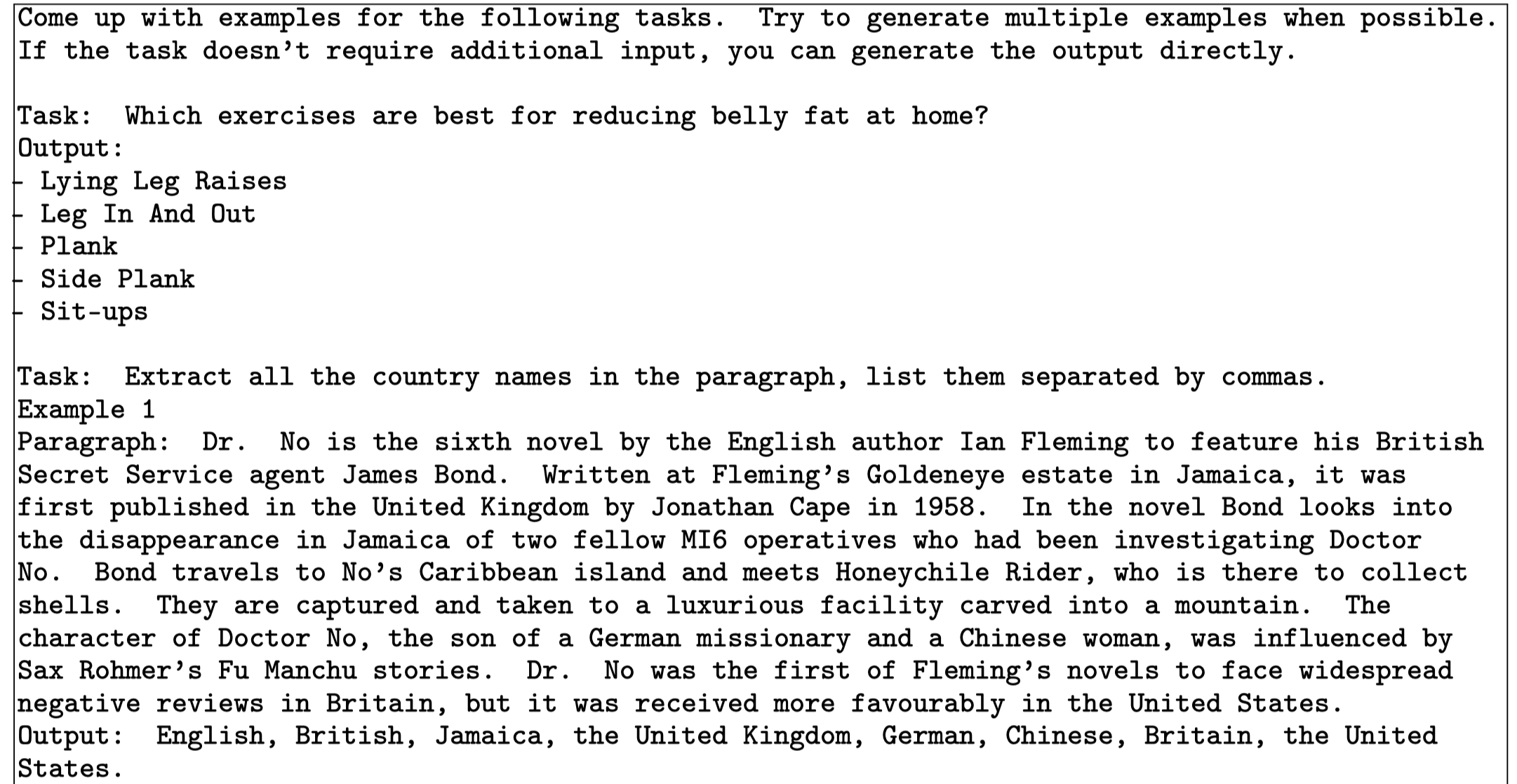
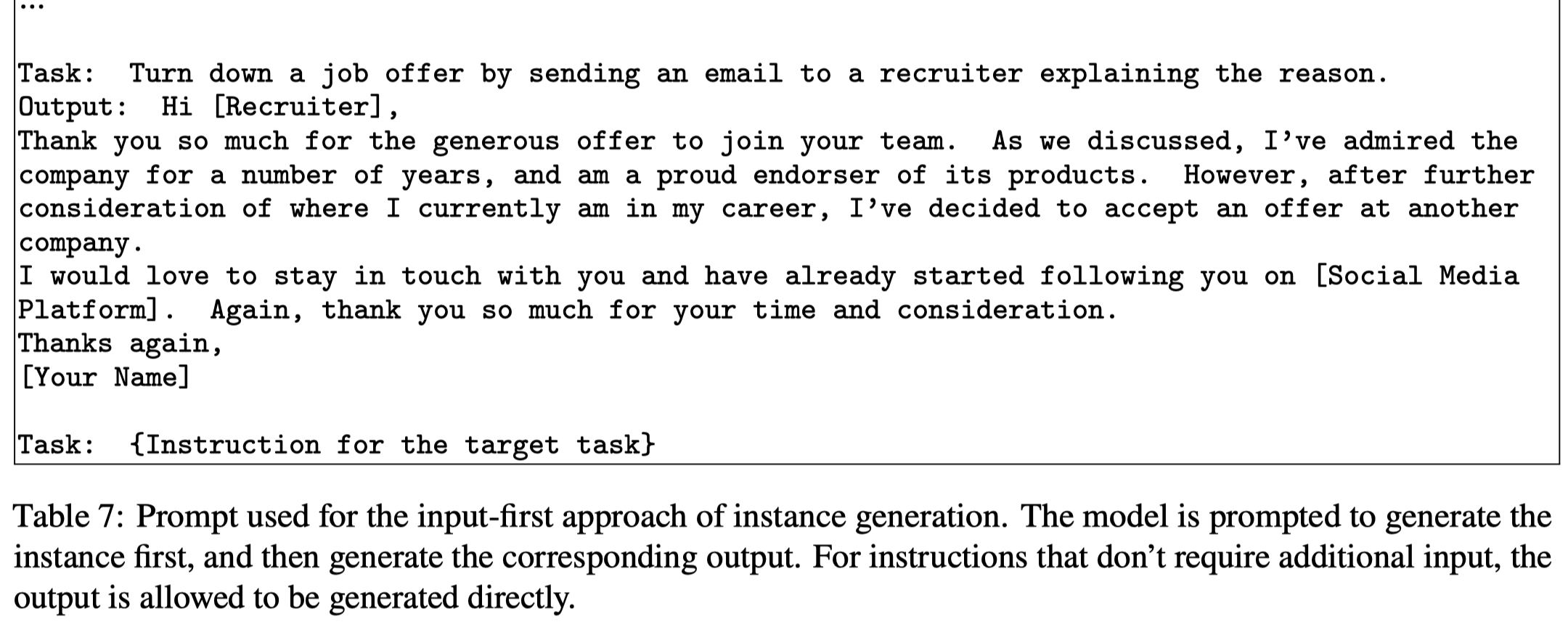
对于分类任务,作者发现如果是先生成input,LLM总是会倾向于生成某一个label的输入。因此作者使用LLM先生成output label,再让LLM生成input,作者称为Output-first Approach:
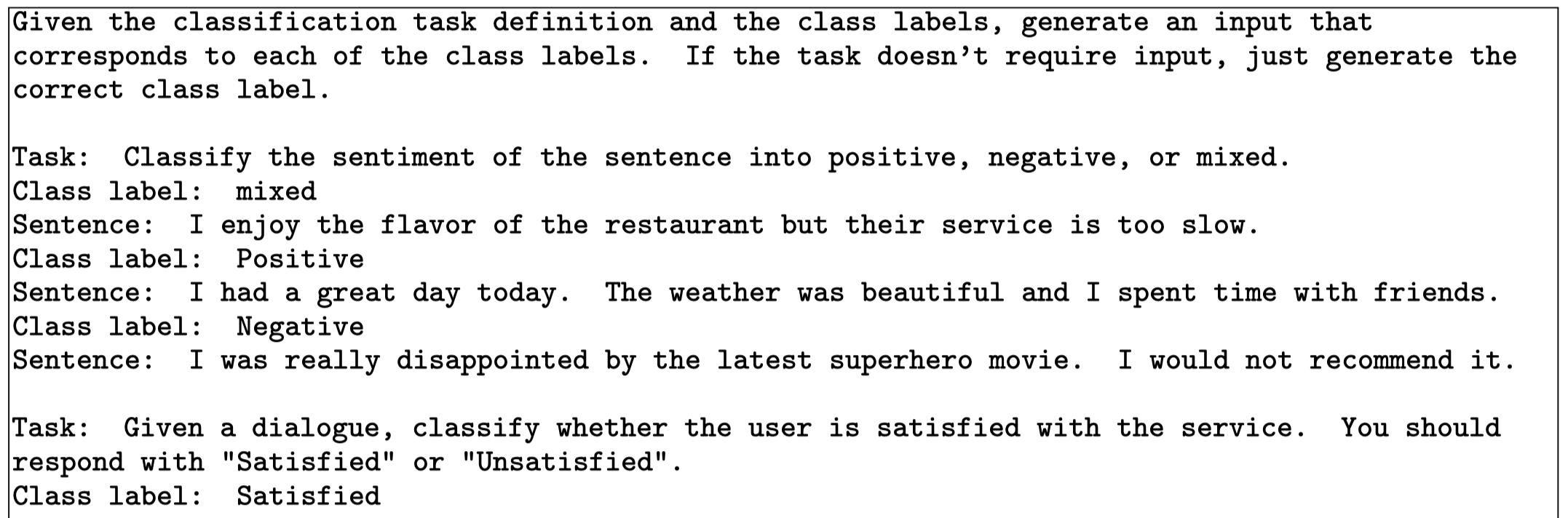
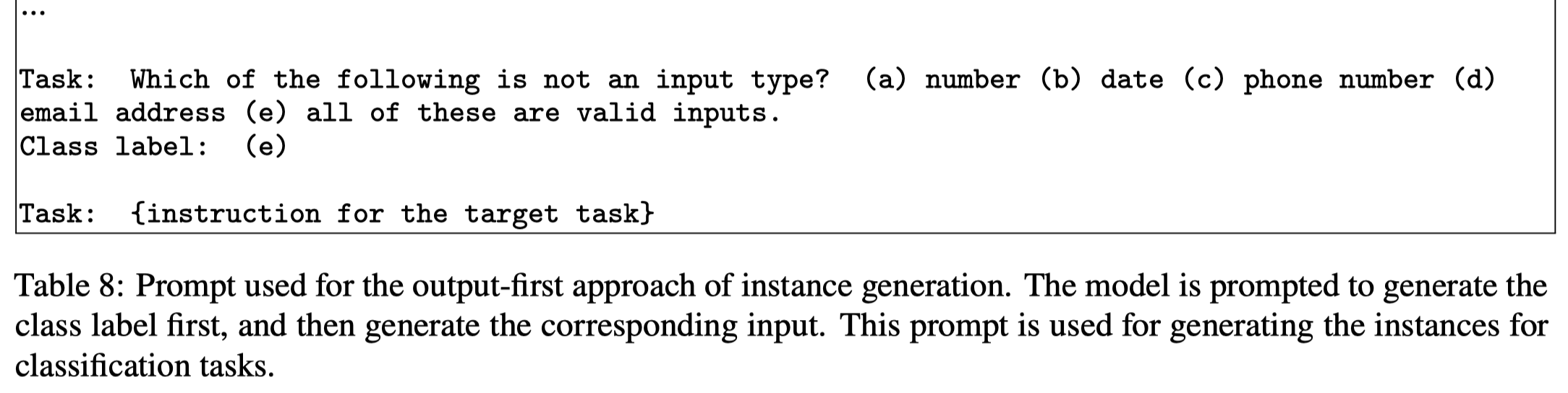
对于LLM生成的task instruction、input和output,需要通过一些规则过滤,比如:
- 只有当和已有的task instruction相似度全部比较低(\(\mbox{ROUGE-L}< 0.7\))的时候,一个新task instruction会被添加到task pool里
- We also exclude instructions that contain some specific keywords (e.g., image, picture, graph) that usually can not be processed by LMs.
- When generating new instances for each instruction, we filter out instances that are exactly the same or those with the same input but different outputs.
- Invalid generations are identified and filtered out based on heuristics (e.g., instruction is too long or too short, instance output is a repetition of the input).
3. Experiment
作者从原始的175个task出发,最后构造了5万多的task,并且差异性也比较大:
不同task instructions的相似度和各种分布统计:
为了进一步确认自动生成的数据的质量,作者随机选择了200个生成的task instruction和对应的1个input-output让作者之一进行人工评估:
可以看出来,自动生成的数据尽管有噪音,还是可以用的,特别是生成的task instructions基本上是现实中成立的说得通的任务。并且那些有错误的样例大多格式是正确的,或者有部分是正确的。
在SuperNI数据集上的实验结果:
SuperNI数据集大多是已有的NLP任务,为了进一步评估模型在实际使用场景下的价值,作者人工创建了一个包括252 task的新数据集。
We first brainstorm various domains where large LMs may be useful (e.g., email writing, social media, productivity tools, entertainment, programming), then craft instructions related to each domain along with an input-output instance.
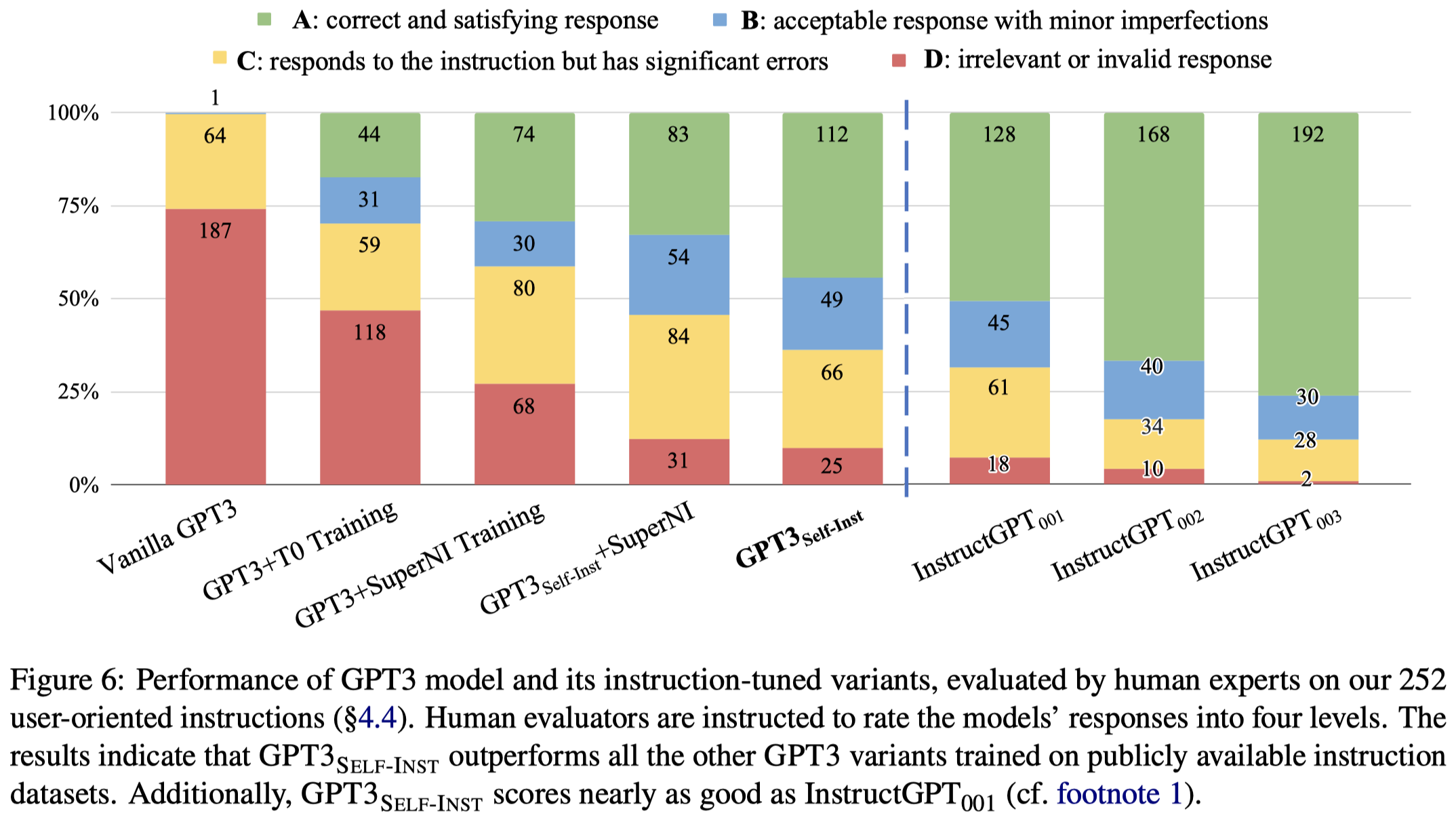
从效果上来看,作者加入self-instruct效果确实好于vanilla GPT-3(davinci),效果接近InstructGPT001(text-davinci-001)。
最后,作者评估了不断加入instructions进行fine-tuning的效果:
图上红色的点是很有趣的,那个红色的结果是作者使用text-davinci-003重新针对每个生成的task instructions重新生成input-output的结果,说明了持续提高instruction-tuning数据质量的效果。